The Generalist Paradigm
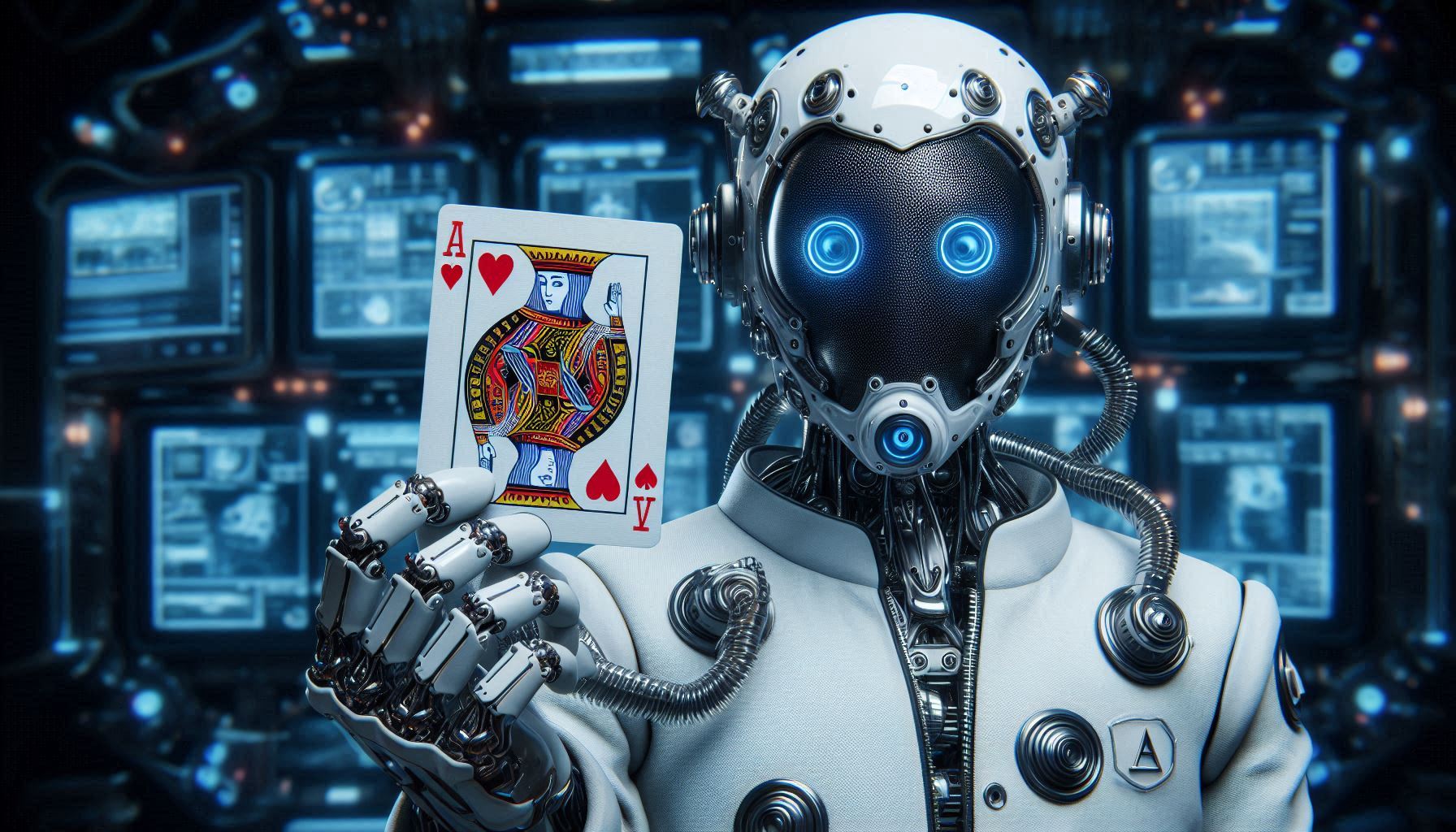
AI agents and large language models (LLMs) are increasingly being used to enhance productivity and time efficiencies. Whether you’re a software developer, accountant, lawyer, author, or from countless other professions, there are benefits from using them for work. In a 2024 survey, about a third of participants use LLMs everyday with close to half using them more than once a week.
Depending on your perspective, these statistics may be staggering. If the history of technology adoption is any indicator - these rates are going to only increase especially when considering that our youngest generations are being exposed to LLMs via popular apps such as Snapchat, Instagram, and others. This will have a familiarity effect including AI as part of these generations’ toolsets as they advance to higher education and eventually join the workforce.
However, large language and attention-based models are not perfect. One example is the need of Mixture-of-Experts to handle specific targeted categories of tasks that showcases a bandaid approach to the diverse variety of work demanded by the consumer. This is due to the unsupervised nature of how these models are trained to scale with vast amounts of uncollated and unorganized data. Therefore, we require specialist models that are domain specific, fine-tuned or trained from scratch.
The generalist model is the allegorical “jack of all trades” and it has downsides. Anyone that has used the current generation (and in my estimation the next couple) of LLMs realizes they excel at information retrieval based on the training corpora or context-based retrieval augmented generation (RAG).
However, when we focus on content generation, we can clearly see that truly creative and innovative outputs are few and far between. If you have access to parameters, you may alter the temperature, measure the perplexity or even execute modified Torrance Tests of Creative Thinking (we are only getting started in this field of research of measuring creative thinking). It is apparent that these models can enhance and augment existing content but not create novelties.
Does anyone really expect the next Tolkien powered by LLMs? Not yet, at least.
Side note: this is one of the core reasons why synthetic training data generation is excruciatingly challenging. You’re stuck in feedback loops that magnify the inherent weaknesses of these model architectures. Conclusively, LLMs are permutators of existing data.
As LLMs become more widely adopted by professionals, it could paradoxically lead to a decline in innovation. The challenge is that automating processes that require human creativity and ingenuity is not possible with the current level of these technologies. This may be due to the quantum nature of human brain function and reproducing that is obviously quite difficult.
Now, let’s put on our oracle hats and forecast the future by observing the long tail consequences of utilizing LLMs.
Many are calling the rise of LLMs the new “Gutenberg press” event. When the Gutenberg press arrived, the primary benefit to society was clear. Knowledge dissemination via duplicated books allowed for rapid information exchange at levels never seen before.
However, there was also a lesser known hummingbird effect (an innovation in one field leading to breakthroughs/benefits in a different field) in regards to the surging need of eyewear in Medieval Europe. Why? A large part of the population were near-sighted and most didn’t recognize this until reading a book.
If increasing amounts of professionals are utilizing these technologies that clearly have some weaknesses in regards to outputs, what can we expect from the quality of work in the future and what could be the potential hummingbird effects?
We’re already witnessing a number of hummingbird effects:
- Neural implants that augment the brain’s capabilities could defer routine tasks to the implant while enhancing higher-level cognitive processes by freeing up resources to execute them. These could even be AI-powered eyewear for the modern era.
- Quantum computing could be leveraged to address the challenge of true creativity and human ingenuity in AI because of its unique properties. New quantum algorithms intertwined with LLMs are emerging in the industry and will likely continue if we want to tackle the problem and solve it.
- If AI can handle the mundane, repetitive work, it could free us to focus on the more complex tasks. This is a key consideration for the future of work and a competitive advantage that companies need to concentrate on. However, we must be aware of the paradox of innovation.
There is a clear double-edged sword. Practitioners of LLMs that understand the capabilities of these models will reap the most benefits for productivity by acknowledging the strengths and weaknesses of the generalist. By filtering out the hyperbole, the anti-generalist looks to gain the most and this may be where we need to invest for the future.
PS: This article was not written by an AI. That would be the pinnacle of self-defeatism :)